AI in Compliance: A Practical Guide to Real-World Application
- ASD Labs
- Apr 24
- 8 min read
🔑 Key Takeaways
AI helps compliance teams do more – it drafts SARs, summarizes KYC files, and accelerates research, but humans stay in charge.
Compliance automation happens in layers – from summaries to research to decision support, not all at once.
Human-on-the-loop design keeps workflows auditable – every action, prompt, and approval is logged.
You don’t need to rip out your tools – platforms like Chainalysis, Cube3, and Jumio integrate smoothly with AI.
Regulators are signaling support – global institutions expect AI to be part of modern compliance operations.

Why AI Compliance Isn’t a Risk — It’s a Missed Opportunity
Most compliance teams still burn hours writing SARs by hand – even though an LLM could draft a solid first version in seconds.
The tech exists. What’s missing is trust, structure, and a clear plan for implementation.
Whether you’re running a fintech startup or scaling crypto operations, the compliance burden isn’t getting lighter. Manual reviews. Adverse media checks. Endless repetition. It’s not just inefficient – it’s unsustainable.
That’s where AI comes in.
But not in the “replace your analysts” kind of way.
In this article, we’ll show how smart teams are automating compliance workflows without losing control. From drafting summaries to surfacing risk insights to deploying AI agents trained on internal typologies – the tools are already here. And yes, you can build them around KYC processes, audit trails, and team sign-offs.
AI compliance isn’t a black box anymore – it’s software. And with the right architecture, it becomes a force multiplier for your team.
Compliance Needs Relief, Not Reinvention
Compliance teams aren’t short on responsibility — they’re short on hours. From reviewing customer files to preparing SARs and documenting decisions, the volume of manual, repetitive tasks continues to grow. But while the regulatory landscape evolves rapidly, many internal workflows remain stuck in spreadsheets and PDFs.
The result? Burnout, bottlenecks, and mounting risk.
Technology vendors often pitch AI as a cure-all. But for most founders, CTOs, and compliance leads, the pitch feels disconnected from reality. They don’t want to gamble with black-box tools or risk automating sensitive decisions without oversight. What they need is something more grounded — a clear framework for using AI as a force multiplier, not a replacement.
That’s exactly what this article delivers. We'll break down three practical levels of AI integration — from assistant-style tools that help draft KYC summaries, to full-stack systems that detect risk patterns in real time. Each level keeps humans in control, with safeguards and traceability built in.
But before diving into those architectures, let’s zoom out. Why is this shift so important right now?
Because compliance work doesn’t scale the way your business does — and that’s the real problem.
Here’s why that’s a critical shift.
The Bottleneck Problem – Why Compliance Work Doesn’t Scale
Compliance has always been a headcount problem. More customers, more transactions, more jurisdictions — the only way most teams keep up is by hiring. But in today’s market, that model breaks fast.
Even mature fintech and crypto firms struggle to scale their compliance operations. Hiring is expensive. Training is slow. And the work itself? Repetitive, fragile, and prone to human error.
Here are the real constraints slowing teams down:
Manual investigations dominate analyst time
Reviewing adverse media hits, double-checking ownership structures, cross-referencing lists — these are tasks that could be assisted by tools, but usually aren’t.
SARs and narrative summaries are labor-intensive
Drafting a coherent, regulator-ready narrative takes time and judgment. But much of the initial drafting follows patterns AI is perfectly suited to handle.
Audit trails require precision under pressure
Any misstep in documentation can create liability. That’s why analysts double-check everything — even if they’ve done it a hundred times before.
These bottlenecks aren’t just inefficient — they create operational risk. When analysts are stretched thin, they miss signals. They skip checks. The whole process becomes reactive, not proactive.
What’s needed isn’t a larger team — it’s a smarter one. And that starts by changing how we think about automation.
In the next section, we’ll map out the three layers of AI integration that leading teams are already putting into place — starting with the simplest and most accessible one.
3 Levels of AI Integration in Compliance Workflows
AI integration doesn’t need to be all-or-nothing. The smartest compliance teams aren’t replacing their analysts — they’re building around them. What’s emerging is a clear, staged approach that introduces automation in controlled, auditable layers.
Let’s break it down into three practical levels.
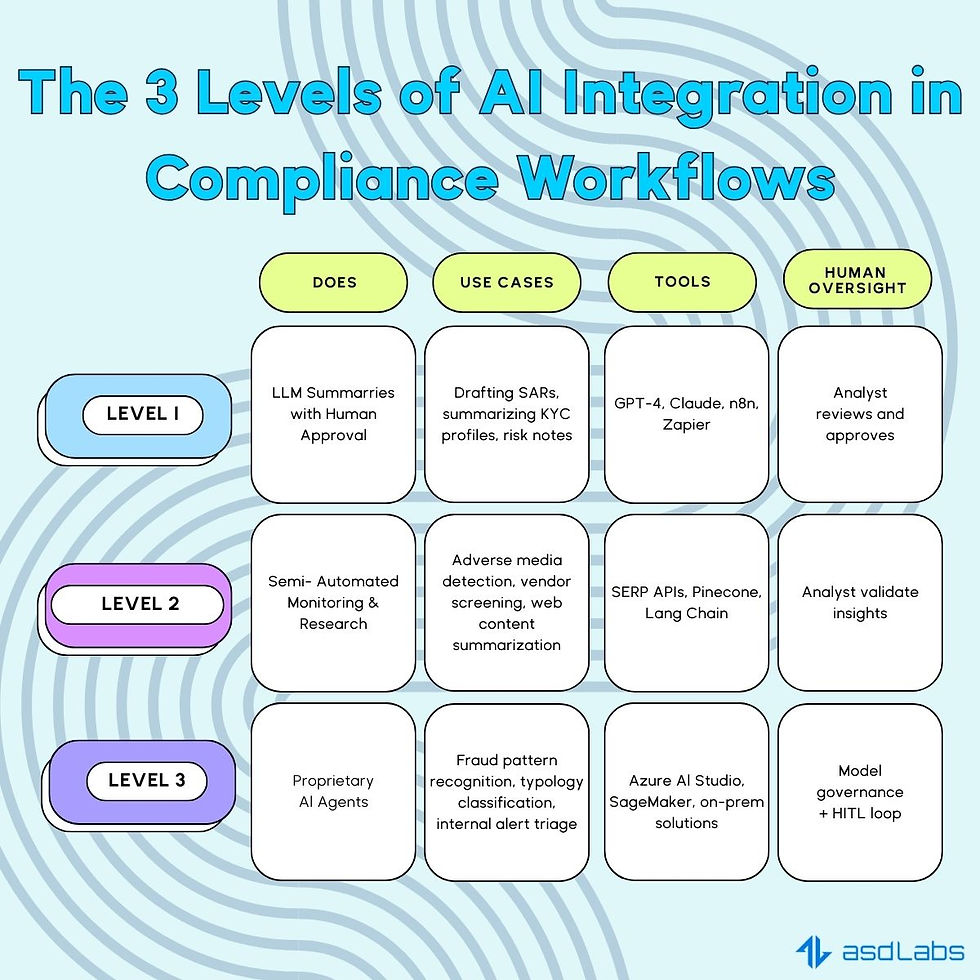
Level 1 – LLM Summaries with Human Approval
This is the entry point for most teams. LLMs like GPT‑4 or Claude are used to draft suspicious activity reports (SARs), KYC file summaries, or risk digest notes. These drafts follow internal formats but save hours of repetitive writing.
How it works:
An orchestration tool (n8n, Zapier) pulls structured data into an LLM prompt
The model generates a first draft
A compliance analyst reviews, edits, and approves the final version
Why it’s safe:
No decisions are made by the model
All inputs and outputs are logged
Prompts are version-controlled and can be adjusted for tone and precision
This level typically reduces drafting time by 70–90% — while keeping oversight exactly where it belongs: with your team.
Level 2 – Semi-Automated Monitoring and Research
At this stage, AI isn’t just writing — it’s surfacing insights. These workflows combine LLMs with SERP tools and vector databases to accelerate tasks like adverse media checks, PEP detection, and vendor due diligence.
What it looks like:
A SERP or web-scraping agent pulls fresh data from trusted sources
The content is embedded into a vector store (e.g., Pinecone)
An LLM retrieves and summarizes relevant findings for analyst review
The analyst validates findings and tags the case for next steps
Key benefit:
Analysts spend less time Googling — and more time making calls that matter.
This level doesn’t eliminate the need for human review — it simply turns two hours of searching into two minutes of reviewing.
Level 3 – Proprietary AI Agents Trained on Internal Data
This is where automation moves from helpful to transformative.
Advanced teams are now using internal SARs, customer behavior logs, and typology datasets to train custom AI agents. These models help detect fraud patterns, flag transaction anomalies, and classify risk — all with minimal human prompting.
Platform examples:
Azure AI Studio
AWS SageMaker
In-house LLM pipelines with domain-specific training
Risk controls required:
Governance frameworks for model training and validation
Audit logs, explainability protocols, version tracking
Human-on-the-loop at critical decision points
This isn’t for everyone. But for large compliance teams dealing with high transaction volumes, Level 3 unlocks scale without sacrificing trust.
For a deeper dive into layered transaction monitoring strategies, explore our detailed guide on crypto compliance frameworks.
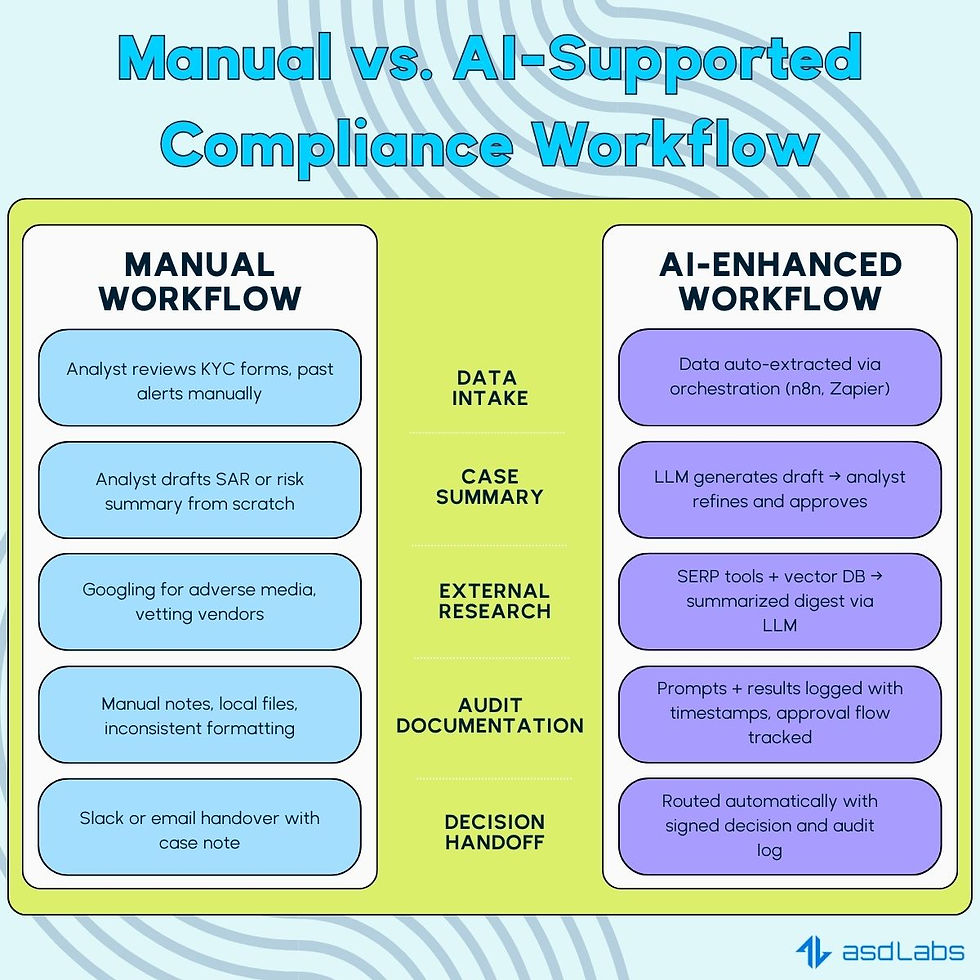
Real Tools in the Stack – From Chainalysis to Cube3 to n8n
One of the biggest misconceptions about AI integration is that it requires replacing your existing systems. In reality, most modern compliance teams are building on top of their current tools — not around them.
Here’s how existing platforms already support AI workflow.
Identity and KYC – Jumio, Veriff, Onfido
Identity verification tools remain foundational — but they generate rich data that can be reused.
LLMs can summarize KYC files before analyst review, flagging gaps or inconsistencies
Orchestration tools like n8n or Zapier can automatically extract relevant metadata for case notes
This reduces manual note-taking and creates more consistent documentation for auditors.
Transaction Monitoring and Typology Detection – Chainalysis, Amlyze, Cube3
These tools already provide alerting and behavioral analytics — but they don’t always surface why a case is interesting.
Use LLMs to generate natural language rationales for flagged transactions
Combine outputs with internal typology models to cluster alerts by risk pattern
This helps analysts triage faster — and makes the review process easier to audit later.
Workflow Automation and Integration – n8n, LangChain, Zapier
These platforms are the glue of modern compliance stacks.
Use n8n to trigger AI summaries after new alerts
Use LangChain to build retrieval pipelines across internal data + vector DBs
Use Zapier to post results into Slack, Notion, or your case management system
The takeaway? You don’t need to wait for “AI-first” vendors. You can start now — using tools your team already knows.
And when AI becomes just another node in your workflow, it starts to feel less risky — and a lot more useful.
Next, we’ll look at how to make those workflows auditable by design — so your team stays in control, even as automation ramps up.
Governance by Design – AI Can Be Controlled, Audited, and Approved
The biggest concern around AI in compliance isn’t performance — it’s accountability.
What happens when a model gets it wrong? Who signs off? Can you trace the decision back?
Smart teams solve this by designing governance into the workflow from day one. That means clear handoffs, visibility into what the model did, and the ability to reverse or re-execute any output.
Here’s what that looks like in practice:
Every Action is Logged
Whether an LLM drafts a SAR or flags a risk cluster, the output isn’t ephemeral.
Prompt versioning ensures you know what the model was asked, when, and by whom
Timestamps and user IDs are attached to every action, whether it's automated or human-reviewed
Audit trails are complete, not partial — every handoff is captured
This gives compliance officers something they’ve rarely had in AI systems: confidence.
Analysts Stay in Control
These workflows don’t bypass human judgment — they route it.
Analysts can edit, approve, or reject LLM outputs
Case management tools track approvals as part of standard review
Automated decisions (e.g., alert prioritization) are always overridable by policy
You define the thresholds. You set the gates. AI acts within that perimeter — not beyond it.
AI That Can Be Audited Is AI That Can Be Trusted
Auditors, regulators, and internal stakeholders aren’t just asking if you use AI — they want to know how. Governance turns that conversation from defensive to strategic.
Done right, your compliance workflow doesn’t just use AI — it proves it used it responsibly.
And if regulators come knocking, you’ll have more than just results.
You’ll have the receipts.
Up next: how regulatory bodies and supranational institutions are already paving the way for AI in compliance — and why that’s a green light, not a red flag.
What Regulators and Institutions Are Signaling
For years, AI in compliance sat in a grey area — promising in theory, risky in practice. Today, that’s changing fast.
From Europe to the U.S., regulators and international bodies are beginning to acknowledge what many compliance leaders already know: smart use of AI is not only acceptable – it’s becoming expected.
Supranational Bodies Are Setting the Tone
Organizations like the FATF, BIS, and IMF have each highlighted the role of emerging technologies in financial crime prevention.
FATF’s Opportunities and Challenges of New Technologies for AML/CFT notes that AI can improve both detection and efficiency — if properly governed
The EU’s AI Act emphasizes transparency and human oversight, not prohibition
Regulatory sandboxes across the UK, Singapore, and Lithuania actively test AI-based solutions in live environments
The message is clear: regulators want to see automation that’s measurable, auditable, and human-centered.
To understand how automation shapes financial systems beyond compliance, consider our analysis of stablecoin adoption and infrastructure.
AI Readiness Is Becoming a Competitive Advantage
Early adopters are already moving.
Fintechs and crypto platforms that build AI-ready stacks are reducing operational costs, improving case throughput, and tightening their audit posture — without expanding headcount.
And when examiners see clear controls, well-documented prompts, and human checkpoints?
That doesn’t raise eyebrows. It raises confidence.
Regulation Is No Longer a Barrier — It’s a Framework
The idea that compliance and innovation are at odds is outdated. The future is collaborative.
AI that respects policy boundaries, enables oversight, and supports transparency fits neatly within most modern regulatory frameworks.
What’s needed now is execution — the kind that scales what your team already does well.
So where does that leave us?
Conclusion – AI Isn’t a Risk to Compliance. It’s a Roadmap to Resilience
AI in compliance isn’t about handing over control — it’s about taking control back.
The pressure on compliance teams is only growing. More data. More regulation. More complexity. But buried inside that challenge is an opportunity: automation that respects your process, augments your team, and scales with your business.
From drafting SARs to monitoring transactions to spotting emerging risk patterns, AI can now handle the tasks that slow your analysts down — without replacing their judgment.And the best part? It doesn’t require a full-stack rebuild. Just the right layers, connected with intent.
The institutions are ready. The tools are mature. The guardrails are already in place.
The only question is whether you’ll wait — or design systems that work better now, while everyone else is still figuring it out.
Start small. Build around your team. And treat AI like what it is — infrastructure, not magic.
Because the future of compliance isn’t less human.
It’s more human – just with better tools.
Comments